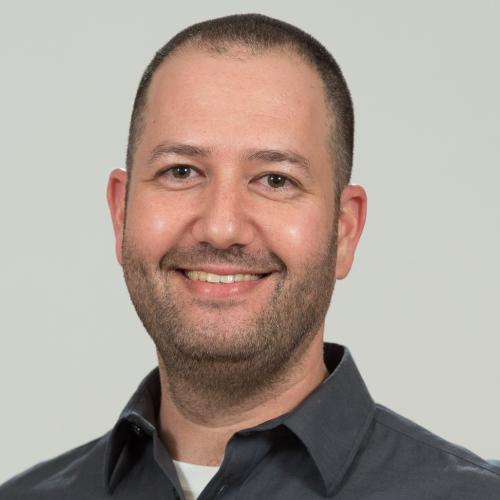
Lab Website
Computational Intelligence, Experimental Optimization, Statistical Learning, Theory of Randomized Search Heuristics, Quantum ML
The advent of modern laboratory and field experiments, as well as of computerized systems, enables researchers to control experiments and analyze their big-data in high-speed rates. Yet, algorithmic design of intelligent experiments for optimization and learning of prescribed objectives may constitute the next level. Very little is known today about the general usefulness of optimization heuristics and statistical learning methods in laboratory experiments, about their strengths and weaknesses when compared to traditional Design-of-Experiments approaches, or about any kind of guidelines which approach to prefer, depending upon the dimensionality of the search-space, the levels of uncertainty and the number of available trials.
We are interested in learning and optimization questions related to systems within the Natural Sciences. In particular, Operational Research and Machine Learning aspects of experimental domains, that is, practical physical- and life-sciences problems whose computerized models are unavailable or too expensive to be executed, and thus necessitate real-world experiments toward the end of their global optimization. Our research interests encompass in general the following topics:
- AI-Driven Scientific Research and Algorithmically-Guided Experimentation
- Artificial General Intelligence, Deep Learning, Self-Supervised Learning
- Combinatorial Optimization (White/Grey/Black-box, including physical systems in the lab)
- Quantum Computing
Our current activities include Experimental Combinatorial Optimization (with Dr. Dan Gamrasni and Dr. Or Shahar), Multi-Objective Mixed-Integer Quadratic Models (with Prof. Michael Emmerich), Effective Detection of Foliage Diseases in Vineyards (with Dr. Rakefet Sharon), and Deep Learning of Enzymes' Functionality (with Dr. Livnat Jurnou and Dr. Itai Sharon).
Education
2008-2010 Postdoctoral Research Associate, Princeton University, USA
2008 Ph.D., Computer Science, Leiden University, The Netherlands
2004 M.Sc., Computer Science, Leiden University, The Netherlands
2003 B.Sc., Physics and Computer Science, The Hebrew University of Jerusalem, Israel
Academic and research positions
2022-2023 Visiting Associate Professor, Faculty of Mathematics, Technion - Israel Institute of Technology
2020- Associate Professor, Computer Science Department, Tel-Hai College
2013- Principal Investigator, MIGAL-Galilee Research Institute
Previously
2019-2022 Head, Computer Science Department, Tel-Hai College
2012-2020 Lecturer and Senior Lecturer, Computer Science Department, Tel-Hai College
2010-2013, Research Staff Member, IBM-Research
- [M.O.S.T.] An innovative AI-driven approach for the development of environmentally-friendly postharvest volatile fungicides (with Dr. Gamrasni/Migal)
- [M.O.A.G.] Innovative AI-driven approach for the development of postharvest protocols (with Dr. Gamrasni/Migal)
- [Migal-internal] Automated identification of enzymes with desirable traits for agriculture use in extreme climate conditions using machine learning (with Dr. I. Sharon and Dr. Afriat-Jurnou)